Probability sampling methods ensure each population member has an equal chance of selection. This approach is vital for achieving accurate and unbiased research outcomes because it is essential for obtaining reliable and generalizable results. This is why probability sampling methods are widely adopted. These methods guarantee equal selection chances, hence, reducing selection bias.
Probability sampling methods, such as simple random sampling and stratified sampling, are preferred in most cases. By employing these strategies, researchers can further ensure their findings reflect the broader population.
Econometrics Tutorials with Certificates
What is Probability Sampling?
Definition and Core Principles
Probability sampling methods are vital in research methodology, enabling the making of statistical inferences about a population. They involve selecting a sample from a population through a random process. That is, this ensures each unit in the population has a known probability of being included in the sample. Such methods also help reduce selection biases, leading to representative samples that accurately mirror the population’s characteristics.
The fundamental principles of probability sampling include random selection, known probabilities of selection, and a sufficiently large sample size for reliable results. Various probability sampling techniques are available to researchers, such as stratified sampling, cluster sampling, and systematic sampling.
Probability sampling is generally more complex and often more expensive than non-probability sampling. It allows for reliable estimates and statistical inferences about the population.
Important Probability Sampling Methods
These methods help minimize selection biases and provide representative samples that accurately reflect the population’s characteristics. Common probability sampling techniques include simple, stratified sampling, cluster sampling, and systematic sampling.
- Simple Random sampling: each individual or participant has an equal probability of selection.
- Stratified sampling: involves dividing the population into homogeneous groups and selecting independent samples from each group
- Cluster sampling: involves sampling groups or clusters of individuals
- Systematic sampling: involves selecting every nth person from the population after an initial random starting point
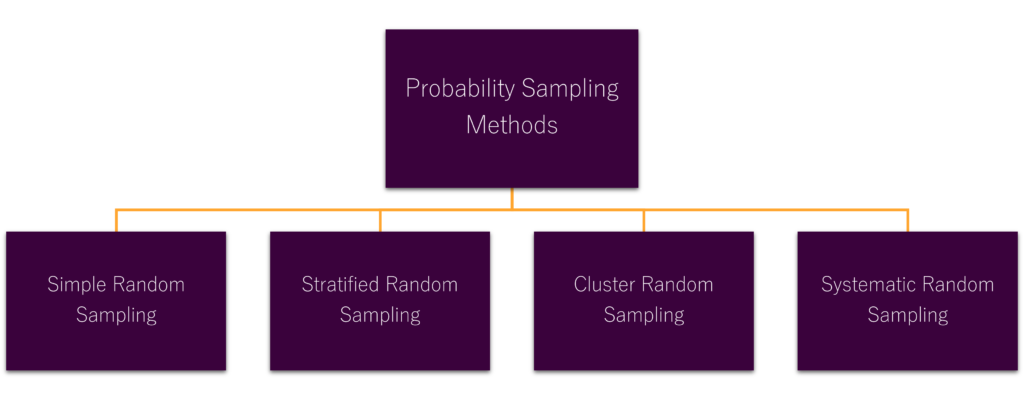
Simple Random, Stratified and Systematic Sampling Techniques
Simple random sampling ensures every population member has an equal chance of selection. It employs randomly generated numbers to pick a sample, making the sampling frame a true reflection of the population. For example, a company might use this method to gauge customer reactions to a new loyalty program by selecting a representative sample from its customer base.
Systematic sampling, by contrast, selects samples through a systematic approach, like every nth item. Hence, this technique guarantees unbiased selection of samples. In market research and customer feedback analysis, these methods are also critical. They provide a fair representation of the population.
Stratified sampling, on the other hand, divides the population into subgroups and participants are selected from each subgroup. That is, stratified sampling involves multiple stages or strata. For example, let us consider a researcher who aims to analyze farm productivity. Using a simple random sampling method might not be ideal because farming productivity could be different for marginal, small and large farmers. Suppose the population has more marginal farmers, then a simple random sampling could lead to a situation that has disproportionately more large farmers than marginal farmers in the sample. As a result, this could skew or bias the results of the study.
Therefore, stratified sampling can be useful in such a situation. The researcher could further divide the farmers into subgroups of marginal, small, medium and large farmers and finally choose random participants from each group. Moreover, the number of participants in the sample from each group may be proportionate or disproportionate to that group’s actual percentage in the population.
Advanced Probability Sampling Approaches
Some advanced methodologies are also instrumental in optimizing the sampling process. This is especially valid in scenarios involving complex populations. As discussed earlier, stratified random sampling divides the population into distinct, homogeneous strata. Then, independent samples are then drawn from each stratum. This method is further advantageous when precision can be enhanced through subgroup analysis. Additionally, we can also use multiple strata or combine it with other sampling methods for complex populations.
Cluster sampling involves segmenting the population into clusters and randomly selecting these clusters for the sample. It is also a practical solution for managing large, geographically dispersed populations. Systematic sampling, by contrast, involves selecting individuals at fixed intervals, starting with a random selection. Moreover, the selection of a probability sampling method is contingent upon the population’s characteristics and the research objectives.
Advanced probability sampling techniques exhibit distinct characteristics:
- Stratified sampling: divides the population into subgroups based on relevant characteristics
- Cluster sampling: selects clusters randomly from a population spread over a large geographical area
- Multistage sampling: combines multiple probability sampling methods to achieve enhanced randomization and minimize biases
Sampling Method | Description |
---|---|
Stratified Sampling | Divides the population into subgroups based on relevant characteristics |
Cluster Sampling | Selects clusters randomly from a population spread over a large geographical area |
Multistage Sampling | Combines multiple probability sampling methods to achieve enhanced randomization and minimize biases |
Applications and Best Practices in Different Fields
Probability sampling methods are extensively applied across multiple domains, including market research, scientific inquiry, and social sciences. Within market research, techniques like simple random sampling and systematic sampling are employed. Hence, these methods aim to gather data that mirrors the population’s diversity. This also ensures that each individual has a fair chance of being selected, leading to a representative sample.
In scientific research, methods such as stratified random sampling and cluster sampling are also utilized to achieve precise outcomes. These strategies further ensure that the sample accurately reflects the population. Hence, it bolsters the reliability and accuracy of research findings.
Conclusion
The choice of a suitable probability sampling method is essential for conducting thorough and dependable research studies. Probability sampling guarantees that every individual in the target population has a known, non-zero chance of selection. This approach also significantly minimizes the risk of sampling bias. The selection of a sampling technique must align with the study’s objectives, the population’s characteristics, and the resources available.
The primary probability sampling methods include simple random sampling, systematic sampling, stratified sampling, and cluster sampling. Each method offers distinct advantages and disadvantages. Researchers must weigh the trade-offs between representativeness, feasibility, and generalizability to choose the most appropriate method for their study. Employing probability sampling techniques, such as the fishbowl draw or random number generators, can enhance the study’s reliability and validity.
Econometrics Tutorials with Certificates
This website contains affiliate links. When you make a purchase through these links, we may earn a commission at no additional cost to you.