The Least Squares Dummy Variables or LSDV approach is one of the ways to estimate the Fixed Effects Model. Hence, the Fixed Effects Model is sometimes called the Least Squares Dummy Variable (LSDV) Model. The Fixed Effects or LSDV model applies to panel data. Panel data contains information over different time periods for separate cross-sectional units. That is, it is a combination of cross-sectional and time-series data.
For instance, suppose we have different individuals’ consumption and income data over time. It will be considered panel data because we have multiple cross-sectional units (individuals) and multiple time periods (time-series data on each cross-sectional unit).
This website contains affiliate links. When you make a purchase through these links, we may earn a commission at no additional cost to you.
Econometrics Tutorials
The Basic Idea behind the Fixed Effects Model or LSDV approach
The Fixed Effects Model (LSDV) allows us to introduce intercept dummies in the model. The purpose of these dummies is that they allow each cross-sectional unit to have a different intercept or to incorporate an intercept that changes over time. For example, we have multiple individuals in panel data. These individuals (cross-sectional units) can have different intercepts because they are heterogeneous units or unique entities. Moreover, their intercepts can vary with time.
This variation in intercept can be in terms of cross-sectional units, i.e. each individual entity has a different intercept. It can vary over time periods, i.e. intercept changes over time. Finally, it is possible to have a combination of both individual and time-specific fixed effects.
Specification of the Fixed Effects Model (LSDV approach)
The Fixed Effects model, therefore, uses cross-sectional dummies to account for variations in cross-sectional units and time dummies to account for variations in intercept over time. Let us consider a simple model:

We are dealing with panel data as we have cross-sectional units (i) and time periods (t). Hence, we can introduce cross-sectional and time dummies in this model as follows:
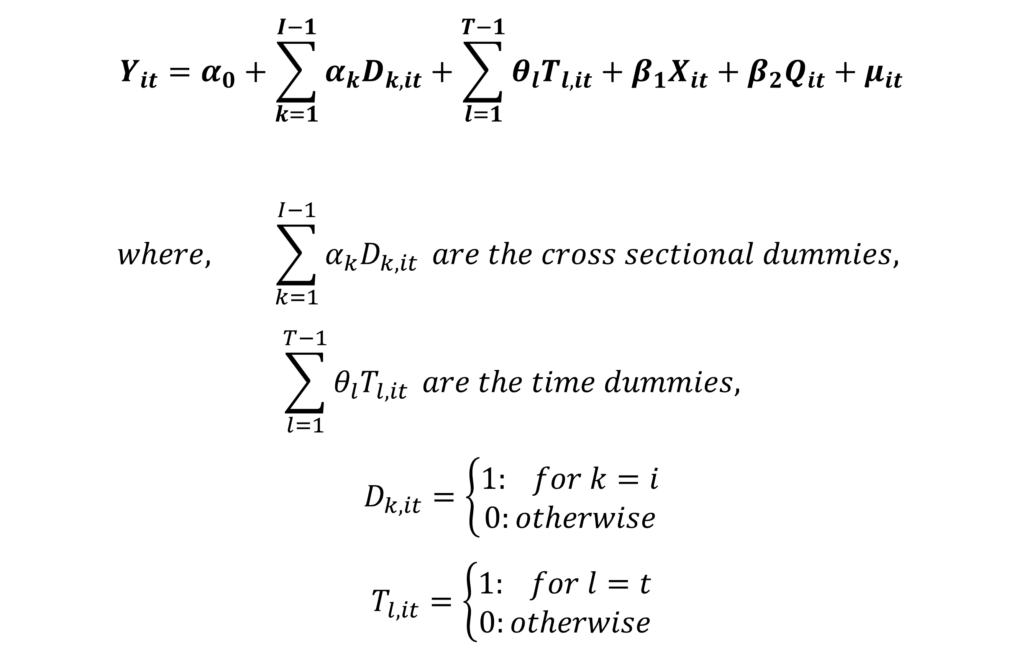
This is what the Fixed Effects Model with the LSDV approach looks like. In the equation, we have a separate intercept for each cross-sectional unit, sometimes called cross-sectional effects. The idea behind this concept is that every unit is heterogeneous, therefore, it does not make sense for all cross-sectional units to have the same intercept. Each unit has its own characteristics which cannot always be measured or included in the model with independent variables. As a result, we need separate intercepts for each unit to capture their unique characteristics or heterogeneity.
Similarly, we have included time-specific effects using the time dummies in the model. That is, each time period has a different intercept to account for any changes across periods that cannot always be measured or included using independent variables.
Avoiding the Dummy Variable Trap
To avoid the dummy variable trap, the number of dummy variables has to be one less than the number of cross-sections and time periods. This means that the number of cross-sectional dummies is equal to “I-1” as shown in the equation above. That is, they are one less than the total number of cross-sectional units or “I”. The number of time dummies is equal to “T-1”, which is one less than the total number of time periods (T).
Suppose we have panel data on 10 individuals over 7 time periods, then we will include 9 cross-sectional dummies and 6 time-specific dummies in the Fixed Effects Model under the LSDV approach. This is necessary because having dummy variables equal to cross-sections or time periods will lead to perfect multicollinearity.
Example
Suppose, we have data on 4 cross-sectional units over 3 time periods. To estimate the Fixed Effects Model, a lot more data is required in practice, but, we are considering these only for illustration purposes. In this case, the Fixed Effects Model can be specified as:
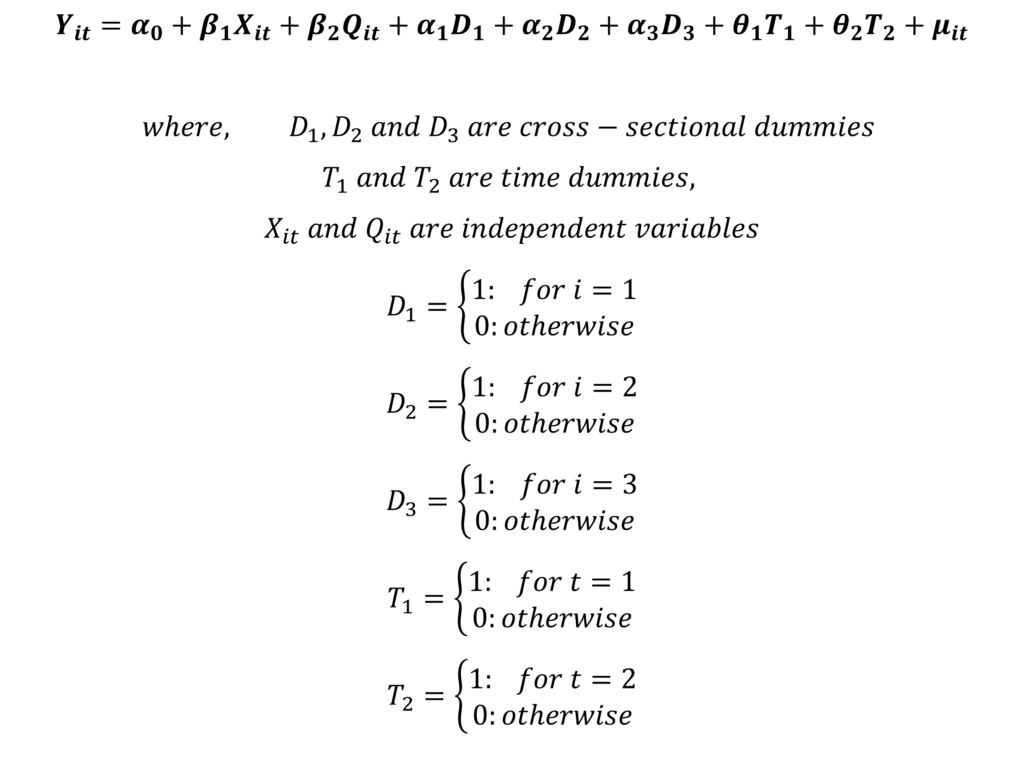
The remaining cross-sectional unit and time period that is not represented with a dummy variable act as the base and we get the estimates for that base when all the dummy variables are zero. We can choose any cross-sectional unit and time period as the base and include dummy variables for the other units and time periods. In this example, we have chosen the 4th cross-sectional unit at the 3rd time period as the base or reference. Hence, the constant “α0” represents the intercept of the 4th cross-sectional unit in the 3rd period.
Similarly, each cross-sectional unit and time period will have a different intercept under this Fixed Effects Model. Some of these intercepts are:
1) α0 ⟹ intercept for 4th unit at period 3
2) α0 + α1 + θ1 ⟹ intercept for 1st unit at period 1
3) α0 + α1 ⟹ intercept for 1st unit at period 3
4) α0 + α3 + θ2 ⟹ intercept for 3rd unit at period 2
… and so on
Before estimation
Before estimating the Fixed Effects Model or LSDV Model, we need to determine whether the cross-sectional or time period effects are significant or not. The Fixed effects model is applicable only when these effects are significant, otherwise, we can estimate the simple model without cross-sectional or time dummies using Pooled OLS.
To test the significance of cross-sectional and time-specific effects, we need to determine whether including the fixed effects in the model significantly improves the model’s fit. That is, we need to determine whether the coefficients of dummy variables are significant using the Wald Test. For Fixed Effects models in general, we can also accomplish this using the F-test for Pooled OLS vs Fixed Effects.
Disadvantages of the Fixed Effects Model
- If we had 15 cross-sectional units, we would have to include 14 cross-sectional dummies. Similarly, if we had 10 time periods, then, we need to introduce 9 time dummies. This means that the number of parameters to be estimated increases with an increase in cross-sections and time periods. This is one of the reasons to be careful when using this model because it can consume a lot of degrees of freedom. The number of dummy variables can be huge when the data has a lot of time periods and cross-sectional units. The results of the model may not be reliable in such cases.
- With a huge number of dummy variables, the possibility of multicollinearity rises which can interfere with the estimation of the model.
- The biggest disadvantage of the Fixed Effects Model is that the fixed effects capture all the heterogeneity of the units. This means that they interfere with the effects of independent variables that do not vary over time. Suppose, we are trying to analyse the impact of independent variables on wages. Some of these independent variables may be gender and ethnicity, which remain the same over time. The dummy variables in the Fixed Effects Model (LSDV) end up interfering with such variables (time-invariant variables) because these characteristics or heterogeneity are absorbed by the dummy variables. This is also true for the Fixed Effects ‘Within’ Estimator even though it does not use dummy variables. The model cannot capture their impact on the dependent variable. Hence, we cannot include time-invariant independent variables in the Fixed Effects Model.
Econometrics Tutorials