Cluster sampling is a probability sampling method that divides a large population into smaller groups known as clusters. This technique is widely used in statistical sampling in various fields, including market research and social sciences. It also allows researchers to focus on specific segments of the population, reducing costs and increasing efficiency in studies.
Cluster sampling is a powerful tool for gathering data from large populations. It involves selecting random clusters from the population, for instance, it can be used to study immunization coverage in a population. As a result, this method has been proven effective in reducing time and resources, making it practical for reaching specific populations. It can also be combined with other methods, like stratified sampling, to enhance the accuracy of results.
Econometrics Tutorials with Certificates
What is Cluster Sampling?
Definition and Basic Concepts
Cluster sampling is a method for data collection from a large population by dividing it into smaller groups or clusters. Additionally, these clusters can be based on geographical location, demographic characteristics, or other criteria. It is commonly used in survey and probability sampling to reduce costs and enhance efficiency. Each cluster serves as a subgroup for detailed study, hence, facilitating a deeper analysis of the population.
The selection of clusters, the sampling frame, and the data collection method are its core components. For instance, in a study on immunization, researchers can employ clusters based on geographical location to gather a representative sample of participants. This technique is further applicable across various fields, including education, market research, and healthcare studies, where random and survey sampling are prevalent.
Key Components of Cluster Sampling
The essential components of cluster sampling are:
- Selection of clusters: This involves randomly selecting a sample of clusters from the population.
- Sampling frame: This is the list of all possible clusters for the study.
- Data collection method: This entails gathering data from the chosen clusters through surveys or interviews.
Types of Cluster Sampling Methods
Cluster sampling is a statistical sampling technique that divides a large population into smaller groups or clusters. Furthermore, it includes single-stage, two-stage, and multi-stage cluster sampling. Each method has its own set of advantages and disadvantages, influenced by the research question, population, and available resources.
Single-stage cluster sampling randomly selects clusters and collects data from all individuals within them. As a result, it is ideal for geographically dispersed populations, yielding substantial samples efficiently. In contrast, two-stage cluster sampling randomly selects clusters first, followed by individuals within those clusters. This approach is, therefore, beneficial for managing sample size while ensuring representativeness.
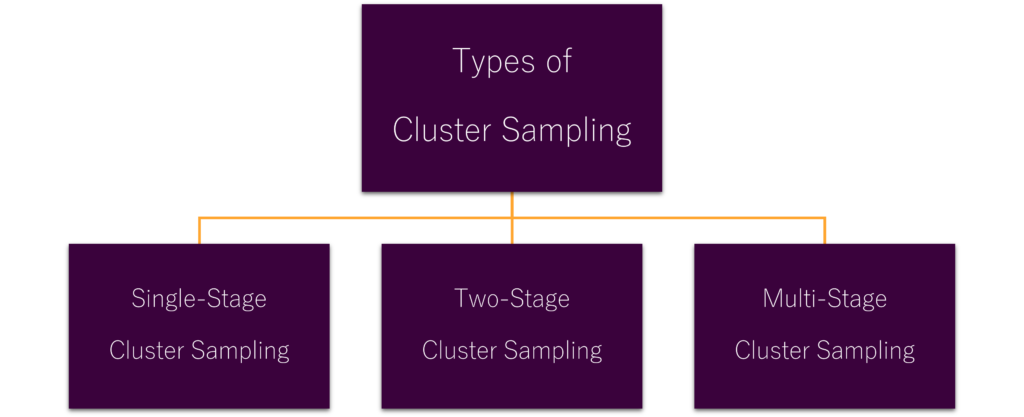
- Single-stage cluster sampling: involves selecting a random sample of clusters and collecting data from every individual in the selected clusters
- Two-stage cluster sampling: involves randomly selecting clusters and then randomly selecting individuals within those clusters
- Multi-stage cluster sampling: involves multiple levels of clustering and is beneficial for studying populations with hierarchical structures
Cluster sampling is a cost-effective method for data collection from large populations. Hence, it is advantageous when complete data collection is impractical due to time or resource constraints. This approach also enhances research data quality and minimizes inaccuracies.
Method | Description | Advantages |
---|---|---|
Single-stage cluster sampling | Randomly select clusters and collect data from every individual | Effective for geographically dispersed populations, provides relatively large samples |
Two-stage cluster sampling | Randomly select clusters and then randomly select individuals within those clusters | Beneficial for managing sample size while maintaining representativeness |
Multi-stage cluster sampling | Involves multiple levels of clustering | Particularly useful for studying populations with hierarchical structures |
Advantages and Disadvantages of Cluster Sampling
Cluster sampling is notable for its cost-effectiveness and efficiency, for instance, it significantly reduces travel costs if the researcher is focusing on geographical clusters. This approach is further ideal for large, dispersed populations, setting it apart from other probability sampling methods. Moreover, it enhances response rates by making subjects within a cluster more accessible. Despite these benefits, it faces challenges such as the risk of bias and the necessity for meticulous planning and execution.
The advantages of cluster sampling include:
- It reduces costs and increases efficiency in data collection, making it beneficial for survey sampling
- It improves response rates due to the accessibility of subjects within a cluster, proving valuable in random sampling
- Also, it allows for insights from a selected cluster, bypassing the need to survey the entire population, which is advantageous in probability sampling
Conversely, cluster sampling’s disadvantages include:
- The risk of introducing bias if selected clusters do not accurately represent the larger population
- Potential for higher sampling errors compared to simple random sampling, leading to underrepresentation of certain groups
- The necessity for careful cluster selection to avoid skewed results that misrepresent broader trends or behaviours within the target population
Real-World Applications and Examples
Cluster sampling is a versatile method, applicable in fields like educational research, market research, healthcare studies, and government surveys. Additionally, it enables researchers to gather representative data from large populations by dividing them into smaller, manageable clusters. For instance, it can be used to study student performance by selecting schools as clusters and surveying all students for educational research. Market research also benefits from cluster sampling, allowing businesses to survey specific geographical regions for more targeted insights into customer preferences and satisfaction.
Some of the examples and key applications include:
- Educational research: to investigate educational outcomes and the effectiveness of teaching methods
- Market research: to identify customer trends and preferences in specific geographical areas
- Healthcare studies: to assess community health quickly by surveying selected neighbourhoods
- Government surveys: to estimate population characteristics and trends by analyzing selected clusters of households
Hence, it is invaluable for handling large and geographically dispersed populations as it simplifies fieldwork logistics and makes studies more manageable.
Comparing Cluster Sampling with Other Sampling Methods
Cluster sampling divides the population into clusters and randomly selects some for the sample. Also, it’s often compared to stratified and simple random sampling. Stratified sampling divides the population into subgroups and samples each subgroup randomly. Simple random sampling, by contrast, selects a random sample from the entire population.
Researchers must weigh the research question, population, and resources when selecting a sampling method. Cluster sampling is cost-effective and also ideal when individual sampling is impractical or too expensive. However, it might be less precise than stratified sampling, which ensures diverse groups are equally represented. Simple random sampling offers strong external validity but can be expensive and time-consuming.
Cluster, stratified, and simple random sampling each have unique strengths and weaknesses. Therefore, understanding these differences enables researchers to select the most suitable method for their study, ensuring accurate and reliable results.
Sampling Method | Advantages | Disadvantages |
---|---|---|
Cluster Sampling | Cost-effective, operationally efficient | May be less accurate than stratified sampling |
Stratified Sampling | Improves precision, reduces errors | Can be time-consuming and expensive |
Simple Random Sampling | Allows for strong external validity | Can be expensive and time-consuming |
Conclusion
Cluster sampling emerges as a versatile methodology which is also applicable in diverse research domains, from educational research to government surveys and market research. It presents a cost-effective and efficient means of data acquisition and is significantly beneficial for populations spread across vast geographical areas.
The merits of cluster sampling are widely acknowledged. Through meticulous planning and execution of its strategies, researchers can gain profound insights into consumer preferences, behaviours, and other demographic-specific data.
Despite its numerous benefits, it is imperative to be cautious of sampling biases inherent in cluster sampling. By integrating sophisticated statistical methodologies and a strategic approach, researchers can also fully harness the capabilities of cluster sampling. As a result, this enables the delivery of critical insights, facilitating informed decision-making processes.
Econometrics Tutorials with Certificates
This website contains affiliate links. When you make a purchase through these links, we may earn a commission at no additional cost to you.