- You must enroll in this course to access course content.
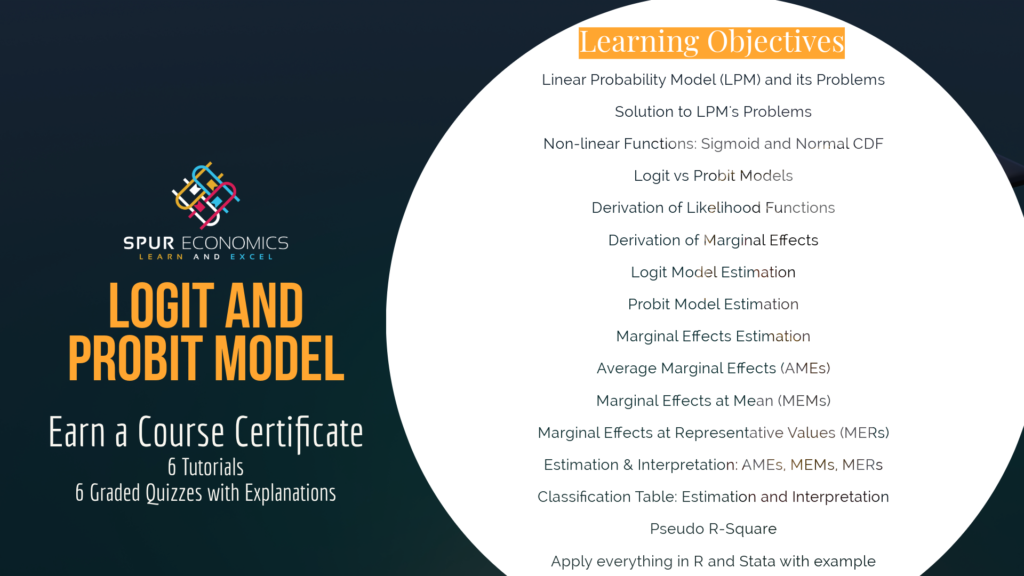
Certificate Sample
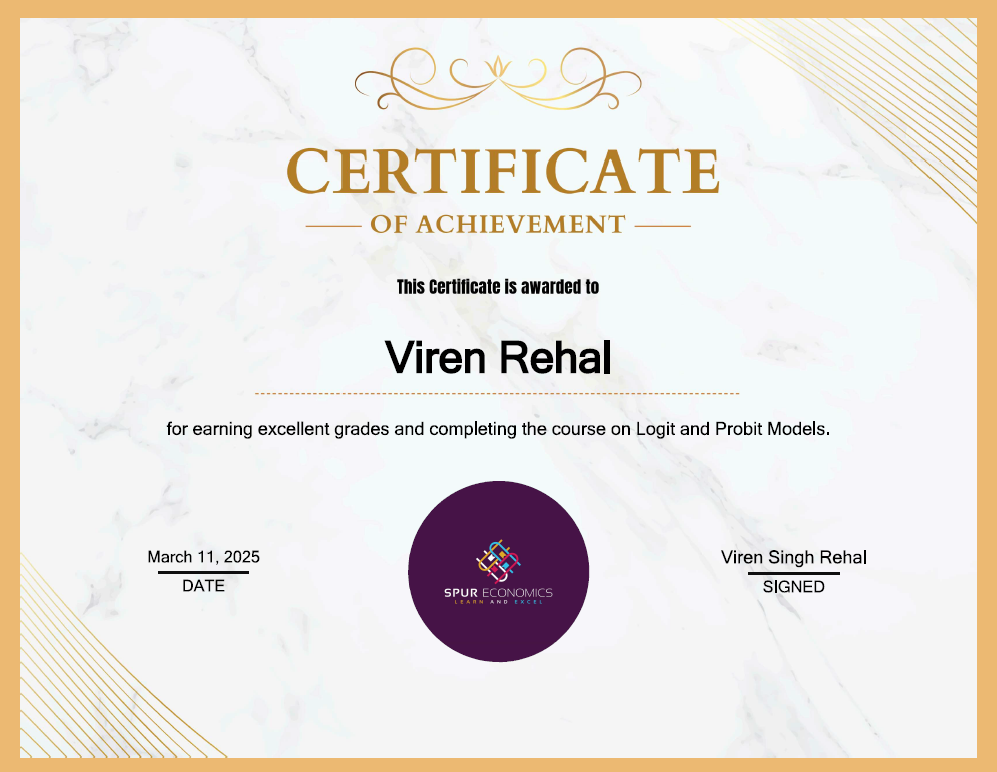
Snippets
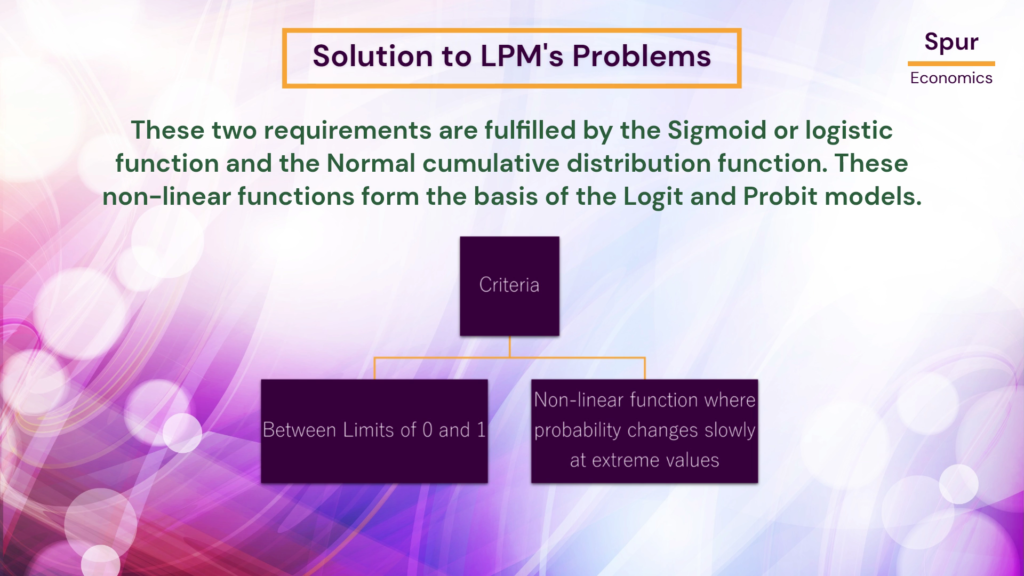
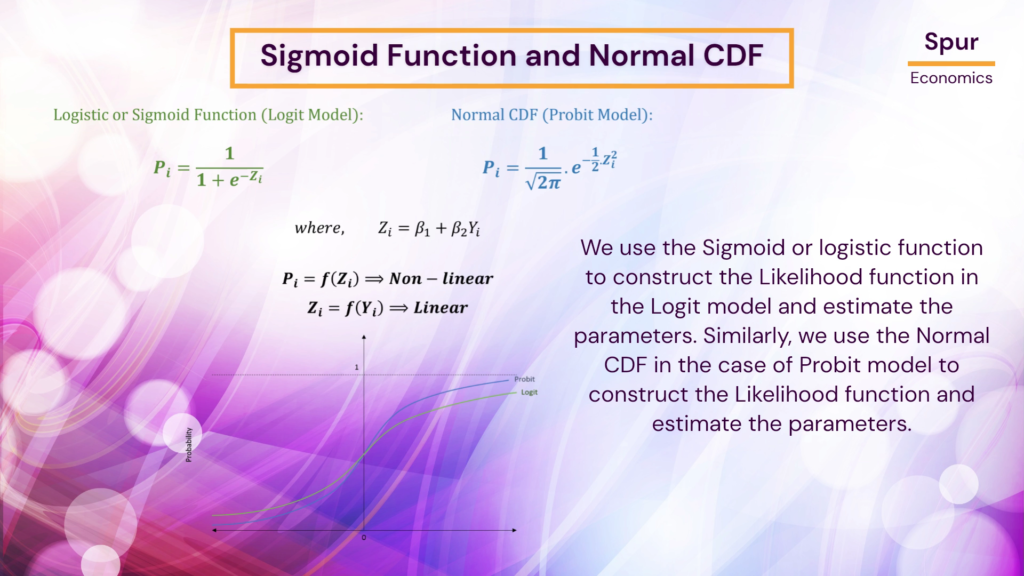
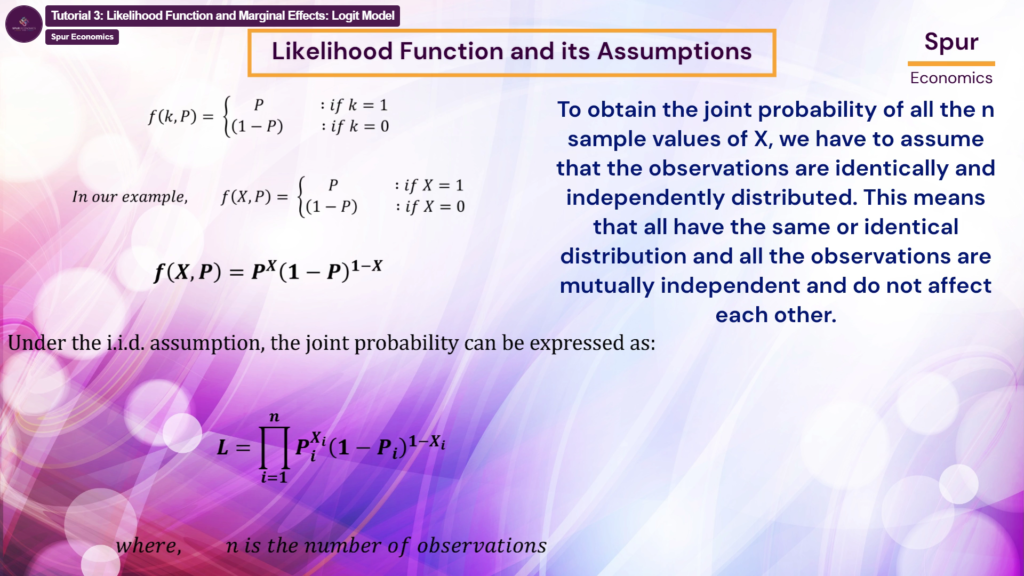
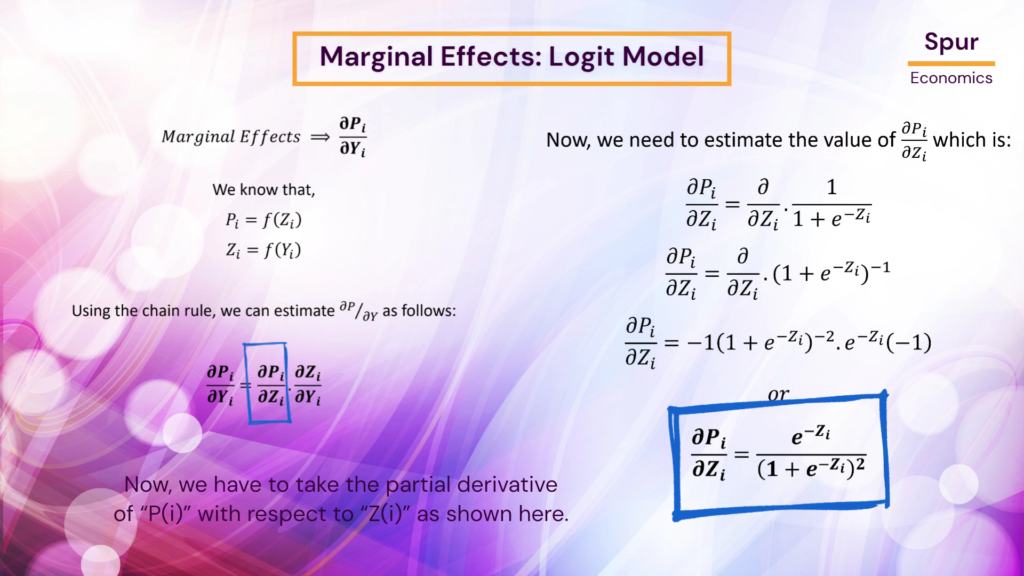
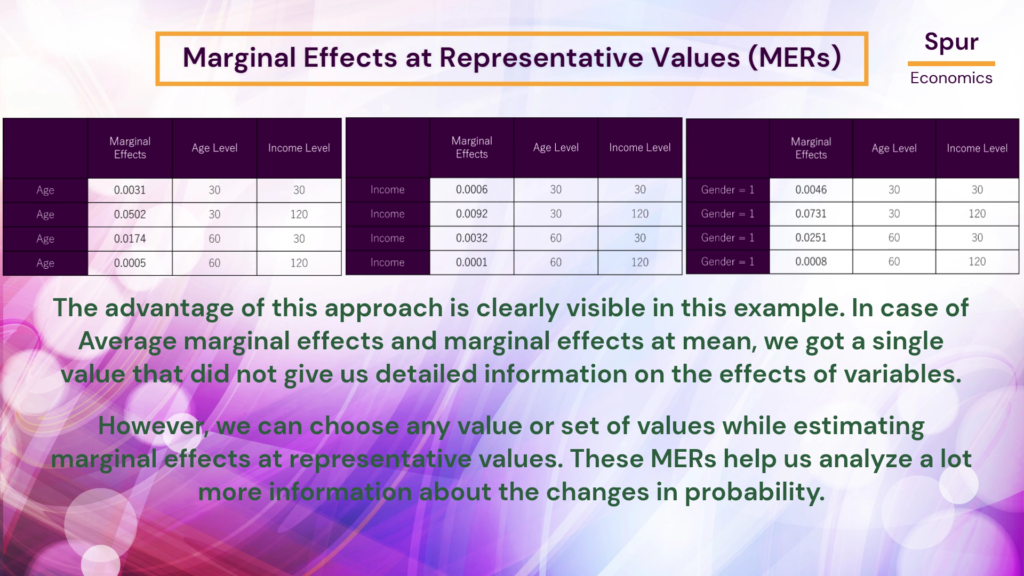
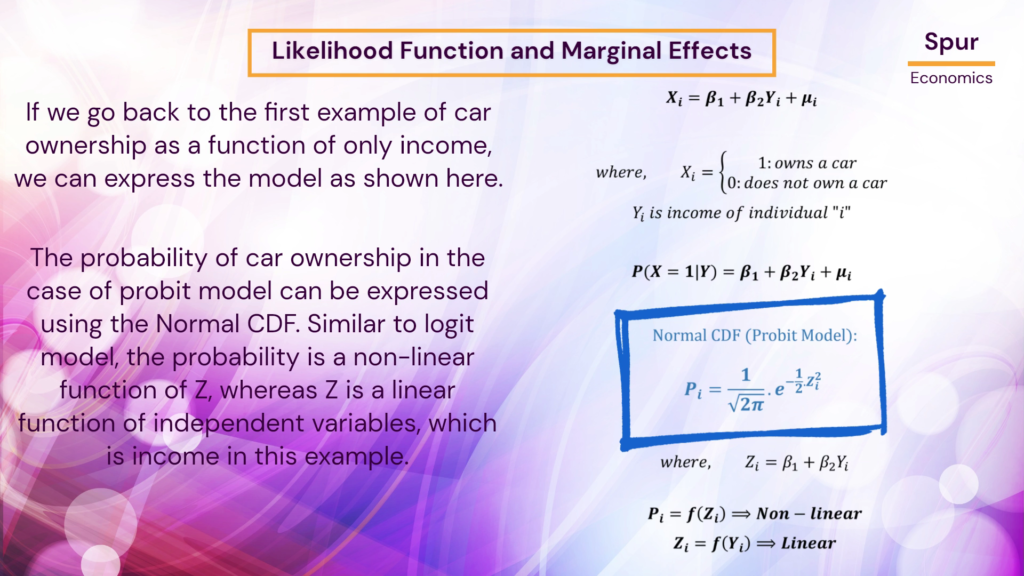
Description
The objective of this series of tutorials is to make the theory and application of Logit and Probit Models easier to understand. The tutorials discuss the important concepts related to Logit and Probit models in detail using suitable examples and quizzes. The tutorials cover the following topics in detail:
- Linear Probability Model or LPM
- Problems associated with LPM: violation of probability limits, heteroscedasticity and linearity assumption
- Why do we need Non-linear functions for binary dependent variables?
- Sigmoid function for Logit Model
- Normal Cumulative Distribution Function for Probit Model
- Logit vs Probit Models
- Likelihood and log-likelihood functions for Logit and Probit models
- Derivation of likelihood functions for Logit and Probit Models
- Marginal Effects after Logit and Probit Models
- Why do we need to estimate Marginal Effects
- Marginal Effects derivation for Logit Model
- Logit Model Estimation in R and Stata
- Probit Model Estimation in R and Stata
- Average Marginal Effects (AMEs) and their estimation in R and Stata
- Marginal Effects at Mean (MEMs) and their estimation in R and Stata
- Marginal Effects at Representative Values (MERs) and their estimation in R and Stata
- Goodness of fit: Classification Table, its meaning and estimation
- Pseudo R-Square